A common problem in messaging middleware is that of efficiently matching message topics with interested subscribers. For example, assume we have a set of subscribers, numbered 1 to 3:
Subscriber |
Match Request |
1 |
forex.usd |
2 |
forex.* |
3 |
stock.nasdaq.msft |
And we have a stream of messages, numbered 1 to N:
Message |
Topic |
1 |
forex.gbp |
2 |
stock.nyse.ibm |
3 |
stock.nyse.ge |
4 |
forex.eur |
5 |
forex.usd |
… |
… |
N |
stock.nasdaq.msft |
We are then tasked with routing messages whose topics match the respective subscriber requests, where a “*” wildcard matches any word. This is frequently a bottleneck for message-oriented middleware like ZeroMQ, RabbitMQ, ActiveMQ, TIBCO EMS, et al. Because of this, there are a number of well-known solutions to the problem. In this post, I’ll describe some of these solutions, as well as a novel one, and attempt to quantify them through benchmarking. As usual, the code is available on GitHub.
The Naive Solution
The naive solution is pretty simple: use a hashmap that maps topics to subscribers. Subscribing involves adding a new entry to the map (or appending to a list if it already exists). Matching a message to subscribers involves scanning through every entry in the map, checking if the match request matches the message topic, and returning the subscribers for those that do.

Inserts are approximately O(1) and lookups approximately O(n*m) where n is the number of subscriptions and m is the number of words in a topic. This means the performance of this solution is heavily dependent upon how many subscriptions exist in the map and also the access patterns (rate of reads vs. writes). Since most use cases are heavily biased towards searches rather than updates, the naive solution—unsurprisingly—is not a great option.
The microbenchmark below compares the performance of subscribe, unsubscribe, and lookup (matching) operations, first using an empty hashmap (what we call cold) and then with one containing 1,000 randomly generated 5-word topic subscriptions (what we call hot). With the populated subscription map, lookups are about three orders of magnitude slower, which is why we have to use a log scale in the chart below.
|
subscribe |
unsubscribe |
lookup |
cold |
172ns |
51.2ns |
787ns |
hot |
221ns |
55ns |
815,787ns |
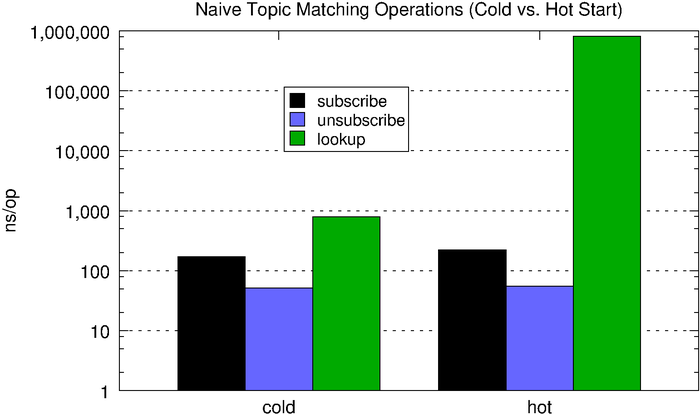
Inverted Bitmap
The inverted bitmap technique builds on the observation that lookups are more frequent than updates and assumes that the search space is finite. Consequently, it shifts some of the cost from the read path to the write path. It works by storing a set of bitmaps, one per topic, or criteria, in the search space. Subscriptions are then assigned an increasing number starting at 0. We analyze each subscription to determine the matching criteria and set the corresponding bits in the criteria bitmaps to 1. For example, assume our search space consists of the following set of topics:
- forex.usd
- forex.gbp
- forex.jpy
- forex.eur
- stock.nasdaq
- stock.nyse
We then have the following subscriptions:
- 0 = forex.* (matches forex.usd, forex.gbp, forex.jpy, and forex.eur)
- 1 = stock.nyse (matches stock.nyse)
- 2 = *.* (matches everything)
- 3 = stock.* (matches stock.nasdaq and stock.nyse)
When we index the subscriptions above, we get the following set of bitmaps:
Criteria |
0 |
1 |
2 |
3 |
forex.usd |
1 |
0 |
1 |
0 |
forex.gbp |
1 |
0 |
1 |
0 |
forex.jpy |
1 |
0 |
1 |
0 |
forex.eur |
1 |
0 |
1 |
0 |
stock.nasdaq |
0 |
0 |
1 |
1 |
stock.nyse |
0 |
1 |
1 |
1 |
When we match a message, we simply need to lookup the corresponding bitmap and check the set bits. As we see below, subscribe and unsubscribe are quite expensive with respect to the naive solution, but lookups now fall well below half a microsecond, which is pretty good (the fact that the chart below doesn’t use a log scale like the one above should be an indictment of the naive hashmap-based solution).
|
subscribe |
unsubscribe |
lookup |
cold |
3,795ns |
198ns |
380ns |
hot |
3,863ns |
198ns |
395ns |
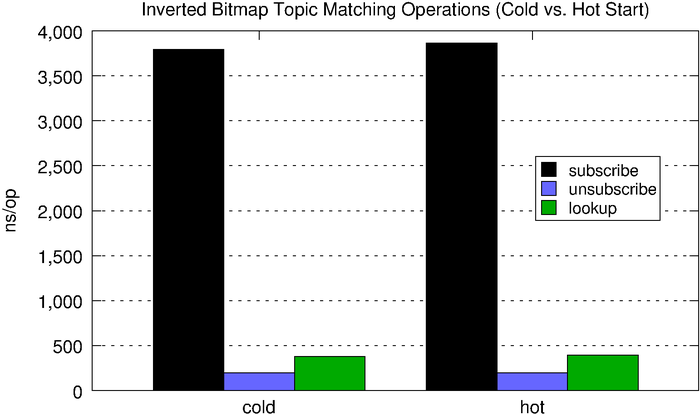
The inverted bitmap is a better option than the hashmap when we have a read-heavy workload. One limitation is it requires us to know the search space ahead of time or otherwise requires reindexing which, frankly, is prohibitively expensive.
Optimized Inverted Bitmap
The inverted bitmap technique works well enough, but only if the topic space is fairly static. It also falls over pretty quickly when the topic space and number of subscriptions are large, say, millions of topics and thousands of subscribers. The main benefit of topic-based routing is it allows for faster matching algorithms in contrast to content-based routing, which can be exponentially slower. The truth is, to be useful, your topics probably consist of stock.nyse.ibm, stock.nyse.ge, stock.nasdaq.msft, stock.nasdaq.aapl, etc., not stock.nyse and stock.nasdaq. We could end up with an explosion of topics and, even with efficient bitmaps, the memory consumption tends to be too high despite the fact that most of the bitmaps are quite sparse.
Fortunately, we can reduce the amount of memory we consume using a fairly straightforward optimization. Rather than requiring the entire search space a priori, we simply require the max topic size, in terms of words, e.g. stock.nyse.ibm has a size of 3. We can handle topics of the max size or less, e.g. stock.nyse.bac, stock.nasdaq.txn, forex.usd, index, etc. If we see a message with more words than the max, we can safely assume there are no matching subscriptions.
The optimized inverted bitmap works by splitting topics into their constituent parts. Each constituent position has a set of bitmaps, and we use a technique similar to the one described above on each part. We end up with a bitmap for each constituent which we perform a logical AND on to give a resulting bitmap. Each 1 in the resulting bitmap corresponds to a subscription. This means if the max topic size is n, we only AND at most n bitmaps. Furthermore, if we come across any empty bitmaps, we can stop early since we know there are no matching subscribers.
Let’s say our max topic size is 2 and we have the following subscriptions:
- 0 = forex.*
- 1 = stock.nyse
- 2 = index
- 3 = stock.*
The inverted bitmap for the first constituent looks like the following:
|
forex.* |
stock.nyse |
index |
stock.* |
null |
0 |
0 |
0 |
0 |
forex |
1 |
0 |
0 |
0 |
stock |
0 |
1 |
0 |
1 |
index |
0 |
0 |
1 |
0 |
other |
0 |
0 |
0 |
0 |
And the second constituent bitmap:
|
forex.* |
stock.nyse |
index |
stock.* |
null |
0 |
0 |
1 |
0 |
nyse |
0 |
1 |
0 |
0 |
other |
1 |
0 |
0 |
1 |
The “null” and “other” rows are worth pointing out. “Null” simply means the topic has no corresponding constituent. For example, “index” has no second constituent, so “null” is marked. “Other” allows us to limit the number of rows needed such that we only need the ones that appear in subscriptions. For example, if messages are published on forex.eur, forex.usd, and forex.gbp but I merely subscribe to forex.*, there’s no need to index eur, usd, or gbp. Instead, we just mark the “other” row which will match all of them.
Let’s look at an example using the above bitmaps. Imagine we want to route a message published on forex.eur. We split the topic into its constituents: “forex” and “eur.” We get the row corresponding to “forex” from the first constituent bitmap, the one corresponding to “eur” from the second (other), and then AND the rows.
|
forex.* |
stock.nyse |
index |
stock.* |
1 = forex |
1 |
0 |
0 |
0 |
2 = other |
1 |
0 |
0 |
1 |
AND |
1 |
0 |
0 |
0 |
The forex.* subscription matches.
Let’s try one more example: a message published on stock.nyse.
|
forex.* |
stock.nyse |
index |
stock.* |
1 = stock |
0 |
1 |
0 |
1 |
2 = nyse |
0 |
1 |
0 |
1 |
AND |
0 |
1 |
0 |
1 |
In this case, we also need to OR the “other” row for the second constituent. This gives us a match for stock.nyse and stock.*.
Subscribe operations are significantly faster with the space-optimized inverted bitmap compared to the normal inverted bitmap, but lookups are much slower. However, the optimized version consumes roughly 4.5x less memory for every subscription. The increased flexibility and improved scalability makes the optimized version a better choice for all but the very latency-sensitive use cases.
|
subscribe |
unsubscribe |
lookup |
cold |
1,053ns |
330ns |
2,724ns |
hot |
1,076ns |
371ns |
3,337ns |

Trie
The optimized inverted bitmap improves space complexity, but it does so at the cost of lookup efficiency. Is there a way we can reconcile both time and space complexity? While inverted bitmaps allow for efficient lookups, they are quite wasteful for sparse sets, even when using highly compressed bitmaps like Roaring bitmaps.
Tries can often be more space efficient in these circumstances. When we add a subscription, we descend the trie, adding nodes along the way as necessary, until we run out of words in the topic. Finally, we add some metadata containing the subscription information to the last node in the chain. To match a message topic, we perform a similar traversal. If a node doesn’t exist in the chain, we know there are no subscribers. One downside of this method is, in order to support wildcards, we must backtrack on a literal match and check the “*” branch as well.
For the given set of subscriptions, the trie would look something like the following:
- forex.*
- stock.nyse
- index
- stock.*

You might be tempted to ask: “why do we even need the “*” nodes? When someone subscribes to stock.*, just follow all branches after “stock” and add the subscriber.” This would indeed move the backtracking cost from the read path to the write path, but—like the first inverted bitmap we looked at—it only works if the search space is known ahead of time. It would also largely negate the memory-usage benefits we’re looking for since it would require pre-indexing all topics while requiring a finite search space.
It turns out, this trie technique is how systems like ZeroMQ and RabbitMQ implement their topic matching due to its balance between space and time complexity and overall performance predictability.
|
subscribe |
unsubscribe |
lookup |
cold |
406ns |
221ns |
2,145ns |
hot |
443ns |
257ns |
2,278ns |

We can see that, compared to the optimized inverted bitmap, the trie performs much more predictably with relation to the number of subscriptions held.
Concurrent Subscription Trie
One thing we haven’t paid much attention to so far is concurrency. Indeed, message-oriented middleware is typically highly concurrent since they have to deal with heavy IO (reading messages from the wire, writing messages to the wire, reading messages from disk, writing messages to disk, etc.) and CPU operations (like topic matching and routing). Subscribe, unsubscribe, and lookups are usually all happening in different threads of execution. This is especially important when we want to talk advantage of multi-core processors.
It wasn’t shown, but all of the preceding algorithms used global locks to ensure thread safety between read and write operations, making the data structures safe for concurrent use. However, the microbenchmarks don’t really show the impact of this, which we will see momentarily.
Lock-freedom, which I’ve written about, allows us to increase throughput at the expense of increased tail latency.
Lock-free concurrency means that while a particular thread of execution may be blocked, all CPUs are able to continue processing other work. For example, imagine a program that protects access to some resource using a mutex. If a thread acquires this mutex and is subsequently preempted, no other thread can proceed until this thread is rescheduled by the OS. If the scheduler is adversarial, it may never resume execution of the thread, and the program would be effectively deadlocked. A key point, however, is that the mere lack of a lock does not guarantee a program is lock-free. In this context, “lock” really refers to deadlock, livelock, or the misdeeds of a malevolent scheduler.
The concurrent subscription trie, or CS-trie, is a new take on the trie-based solution described earlier. Detailed here, it combines the idea of the topic-matching trie with that of a Ctrie, or concurrent trie, which is a non-blocking concurrent hash trie.
The fundamental problem with the trie, as it relates to concurrency, is it requires a global lock, which severely limits throughput. To address this, the CS-trie uses indirection nodes, or I-nodes, which remain present in the trie even as the nodes above and below change. Subscriptions are then added or removed by creating a copy of the respective node, and performing a CAS on its parent I-node. This allows us to add, remove, and lookup subscriptions concurrently and in a lock-free, linearizable manner.
For the given set of subscribers, labeled x, y, and z, the CS-trie would look something like the following:
- x = foo, bar, bar.baz
- y = foo, bar.qux
- z = bar.*
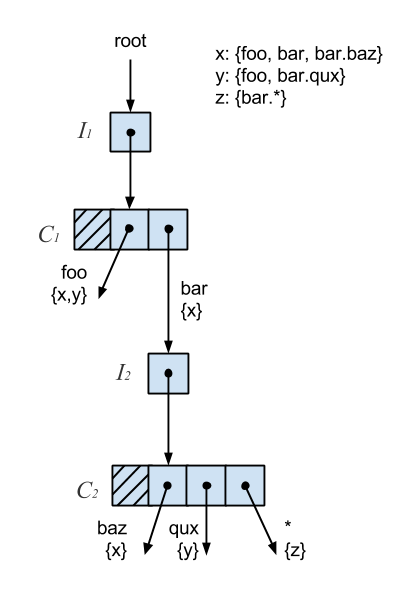
Lookups on the CS-trie perform, on average, better than the standard trie, and the CS-trie scales better with respect to concurrent operations.
|
subscribe |
unsubscribe |
lookup |
cold |
412ns |
245ns |
1,615ns |
hot |
471ns |
280ns |
1,637ns |

Latency Comparison
The chart below shows the topic-matching operation latencies for all of the algorithms side-by-side. First, we look at the performance of a cold start (no subscriptions) and then the performance of a hot start (1,000 subscriptions).


Throughput Comparison
So far, we’ve looked at the latency of individual topic-matching operations. Next, we look at overall throughput of each of the algorithms and their memory footprint.
algorithm |
msg/sec |
naive |
4,053.48 |
inverted bitmap |
1,052,315.02 |
optimized inverted bitmap |
130,705.98 |
trie |
248,762.10 |
cs-trie |
340,910.64 |
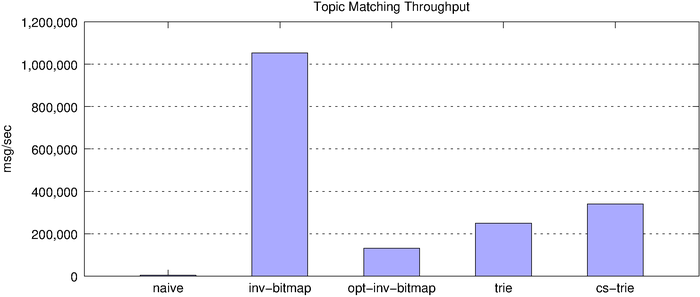
On the surface, the inverted bitmap looks like the clear winner, clocking in at over 1 million matches per second. However, we know the inverted bitmap does not scale and, indeed, this becomes clear when we look at memory consumption, underscored by the fact that the below chart uses a log scale.

Scalability with Respect to Concurrency
Lastly, we’ll look at how each of these algorithms scales with respect to concurrency. We do this by performing concurrent operations and varying the level of concurrency and number of operations. We start with a 50-50 split between reads and writes. We vary the number of goroutines from 2 to 16 (the benchmark was run using a 2.6 GHz Intel Core i7 processor with 8 logical cores). Each goroutine performs 1,000 reads or 1,000 writes. For example, the 2-goroutine benchmark performs 1,000 reads and 1,000 writes, the 4-goroutine benchmark performs 2,000 reads and 2,000 writes, etc. We then measure the total amount of time needed to complete the workload.

We can see that the tries hardly even register on the scale above, so we’ll plot them separately.

The tries are clearly much more efficient than the other solutions, but the CS-trie in particular scales well to the increased workload and concurrency.
Since most workloads are heavily biased towards reads over writes, we’ll run a separate benchmark that uses a 90-10 split reads and writes. This should hopefully provide a more realistic result.
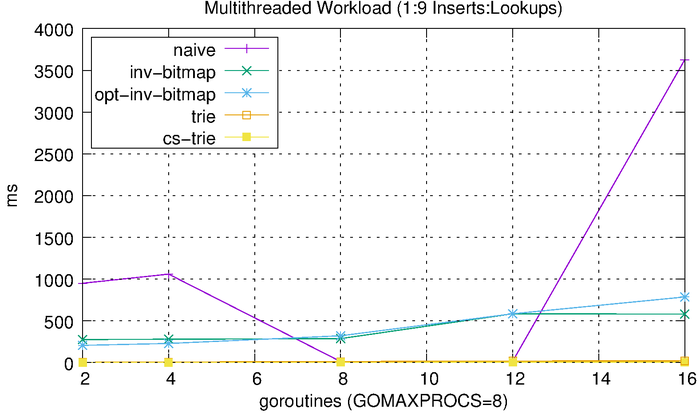
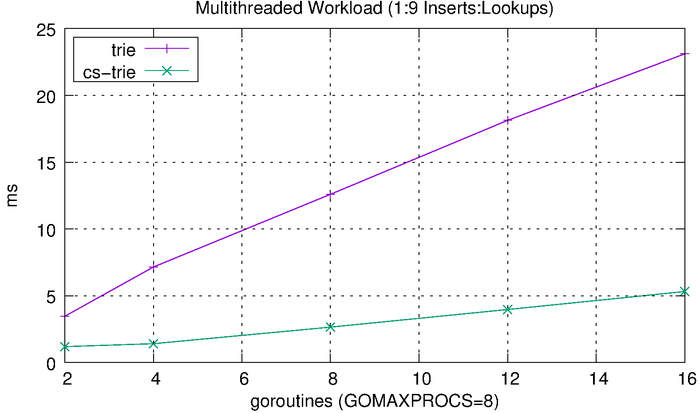
The results look, more or less, like what we would expect, with the reduced writes improving the inverted bitmap performance. The CS-trie still scales quite well in comparison to the global-lock trie.
Conclusion
As we’ve seen, there are several approaches to consider to implement fast topic matching. There are also several aspects to look at: read/write access patterns, time complexity, space complexity, throughput, and latency.
The naive hashmap solution is generally a poor choice due to its prohibitively expensive lookup time. Inverted bitmaps offer a better solution. The standard implementation is reasonable if the search space is finite, small, and known a priori, especially if read latency is critical. The space-optimized version is a better choice for scalability, offering a good balance between read and write performance while keeping a small memory footprint. The trie is an even better choice, providing lower latency than the optimized inverted bitmap and consuming less memory. It’s particularly good if the subscription tree is sparse and topics are not known a priori. Lastly, the concurrent subscription trie is the best option if there is high concurrency and throughput matters. It offers similar performance to the trie but scales better. The only downside is an increase in implementation complexity.